Abteilung Numerical Analysis, Optimization and Scientific Machine Learning
Mathematical models of scientific and engineering processes are rarely solvable in closed form. Thus, the central focus of Numerical Analysis is the development and analysis of efficient methods to compute approximate solutions fast and accurately. Crucially, this also includes the assessment and analysis of the associated errors to correctly interpret the results. Closely related with this topic are the questions of Optimization and Control, as well as the calibration of the mathematical models based on experimental data.
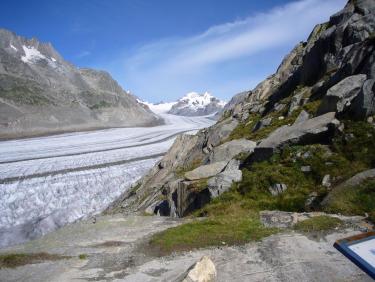
In Heidelberg, the particular focus lies on simulating and optimizing models that are described by systems of ordinary or partial differential equations. The primary concern in Numerical Analysis is the development and analysis of efficient discretization methods, especially of finite element, multiscale and multilevel methods for coupled systems of heterogeneous differential equations. A central aspect in this context is the development of software providing efficient implementations of the developed methods, see also Scientific Computing. Moreover, the associated Uncertainty Quantification is a topic of increasing interest. Here, the focus in Heidelberg lies again on multilevel-/multiscale-methods, as well as on Machine Learning techniques.
Core research areas within Optimization at Heidelberg include methods of smooth and non-smooth optimization also in infinite-dimensional spaces, particularly in the context of differential equation models. Problem classes of specific interest are optimal control, parameter estimation, shape optimization and optimal experimental design, whereby the interplay with the particular choice of discretization plays a central role for the efficient implementation and analysis.
The entire research area distinguishes itself through particularly close, interdisciplinary collaborations with researchers from numerous other departments and faculties at Heidelberg University.